Early-stage lung cancer detection from radiomics to deep learning in thoracic CT images: a narrative review with contemporary clinical recommendations
Introduction
Worldwide, lung cancer is a malignant tumor with a high morbidity and mortality (1). Computed tomography (CT) screening, diagnosis and treatment in the early stage of lung cancer are one of the important means to reduce its mortality (2,3). Lung cancer screening with low-dose CT (LDCT) allows diagnosis and treatment of early stage lung cancer. For diagnosed early stage lung cancer, surgical resection is the preferred treatment, but even after complete resection, there is still considerable heterogeneity in the survival rates. The prediction accuracy of the TNM staging and other traditional prognostic assessment models still need to be improved. Importantly, an accurate assessment of the nature of early stage lung cancer and the prognosis of those patients provide a basis for decision making with respect to the clinical treatment, thus improving the survival of lung cancer patients.
CT images contain important clinical image data that is helpful throughout the diagnosis, treatment and in the prognosis assessment of early stage lung cancer. For example, the National Lung Screening Trial (NLST) found that Screening with the use of low-dose CT reduces mortality from lung cancer(4); The Dutch–Belgian lung-cancer screening trial [Nederlands-Leuvens Longkanker Screenings Onderzoek (NELSON)] reported that lung-cancer mortality was significantly lower among those who underwent volume CT screening than among those who underwent no screening (5). CT imaging analysis has evolved from the Mayo model, which was determined using the classical pulmonary nodules (6), to the current radiomics analysis model (7), and now CT image analysis for early stage lung cancer has gradually entered the field of artificial intelligence area. Among these, radiomics refers to the comprehensive analysis of the tumor characteristics by mining a large number of high-dimensional quantitative characteristics of medical images and combining this with statistical models, which is the bridge between medical image analysis and the realization of precision medicine (8-10). The traditional radiomics analysis process mainly includes image acquisition, lesion segmentation, feature extraction, statistical modeling, and the CT radiomics analysis involves a lung cancer property analysis, a lung cancer gene expression prediction, prognosis assessment, and treatment decision guidance (11-13).
Recently, combined with the advantages of artificial intelligence deep learning (DL), researchers have proposed a new radiomics analysis technology (14,15), namely convolutional neural network (CNN). Because of CNN’s strong image processing capability, it has been successfully applied in the analysis of chest X-ray, CT, MRI and digital pathological images and has assisted clinicians in the diagnosis of diseases, tumor recognition, gene mutation analyses, immunotherapy evaluation, etc. (16). The application of CNN improves the ability of CT radiomics analyses and provides a technical basis for further evaluating the nature and prognosis of early stage lung cancer. Currently, for the assessment of the nature of early stage lung cancer, the detection of pulmonary nodules, the determination of benign and malignant diseases, and the assessment of invasiveness have all been reported in the literature and show advantages over traditional radiomics analyses, which is expected to reach the level of clinicians. However, the results of these nodule characterization studies have not been fully evaluated in clinical practice, and whether DL technology can be applied to the prognosis assessment and treatment decision of early stage lung cancer remains to be explored.
Knowledge of the progress of CT in management of detected lung nodules, as well as the potential developing implication, is essential for thoracic surgeons. This article will describe the research in determining the nature of pulmonary nodule, the prognosis assessment of early stage lung cancer, challenges of CT radiomics analyses in early stage lung cancer and DL and CNN. We present the following article in accordance with the Narrative Review Checklist (available at: http://dx.doi.org/10.21037/shc-20-81).
Research in determining the nature of incidental pulmonary nodules
Development of benign and malignant pulmonary nodules prediction model
The the Fleischner Radiology Society of America published its first edition of advice on the diagnosis and treatment of pulmonary nodules in 2005 (17). As early as 1997, the Mayo Clinic in the United States established the first model for the analysis of benign and malignant solid pulmonary nodules (6). This model established a logistic regression prediction model that was based on age, smoking status, individual tumor history, nodule diameter, burrs, and location in the upper lobe, which is still used today. Meanwhile, Herder et al. (18) validated the Mayo model and pointed out that there was a risk of the model being underestimated in patients judged to be less malignant. However, when combined with PET-CT results, the accuracy of the malignant determination of the nodules (VA model) was improved. Gould et al. (19) developed a new model that incorporated the quantification of smoking history and an assessment of the cessation time. Meanwhile, Schultz et al. verified the Mayo and VA models and proved that the prediction accuracy of Mayo model was better than the VA model (20). During this period, scholars at home and abroad also established and verified new models based on different population characteristics (21-23). Among these, based on domestic multicentre data, we also established and verified a nomogram model for determining isolated solid nodules (SNs) (24).
With the improvement in the sub-solid nodule (SSN) detection rate, McWilliams et al. first divided pulmonary nodules into SN, SSN and pure ground glass nodule (PGGN) to establish a prediction model (Brock model) (25), and two lung cancer screening test cohorts, with a total of 2,961 people and 12,029 nodules, were included. The model indicated that part-solid nodule (PSN) was a risk factor for lung cancer, with an early stage lung cancer incidence of 6.6% and an SN incidence of 1.1%. This study still underestimated the possibility of SSN malignancy, and thus, the criterion for the benign SSN was a stable follow-up for 2 years, but the malignant SSN needed a longer follow-up due to its inertia (26). The team built different malignant models based on whether the proportion of ground glass opacity (GGO) was greater than 50% in SSNs (27). Similarly, based on the previous multicentre database, we also established and verified a nomogram model that predicted PGGN as a precancerous lesion [including benign and adenocarcinoma in situ (AIS)] (28).
The above studies indicate that the benign and malignant determination of pulmonary nodules is an important focus of the clinical work in thoracic surgery. In addition, with changes in the pulmonary nodule disease spectrum, the simple differentiation of benign and malignant has been unable to meet the needs of clinical work, including resection extension, Lymph node dissection range and adjuvant treatment modality. Especially for the assessment of the properties of persistent SSNs, the CT image features of SSNs are different from those of SNs. Typical features, such as aeration of bronchi, average CT value, and proportion of GGO components, also have important clinical significance. At the same time, in addition to the differentiation of benign and malignant, the degree of infiltration also affects the clinical decision. Therefore, the establishment of a new property evaluation model based on CT image features is the key to an accurate SSN diagnosis and treatment.
Application of radiomics in the analysis of pulmonary nodules
Over the past 10 years, scholars have proposed that a large number of high-dimensional quantitative features can be mined and combined with statistical models to comprehensively classify medical tumor images, namely, radiomics (29,30). This method analyzes the features contained in the image that cannot be observed by clinicians visually, further quantifying the heterogeneity within the tumor, analyzing the clinical characteristics of the tumor, and finally providing guidance for clinical decision-making. The information quantification process of traditional radiomics analysis mainly includes four steps: (I) image acquisition and accurate segmentation of the target lesions; (II) reconstructing the three-dimensional structure of the target lesion and extracting a large number of radiomics quantitative characteristics; (III) feature screening, model establishment and verification with different methods; and (IV) evaluation combined with clinical application.
Radiomics not only realizes the analysis of the nodule properties, but also achieves a higher accuracy compared to traditional imaging diagnosis methods (31). Wu et al. (32) extracted 12 traditional imaging features and 13 imaging features of pulmonary nodules from the CT images of 202 patients. The area under the curve (AUC) of the 12 traditional imaging features was 0.84, and the AUC of the 10 imaging features selected by the logistic regression was increased to 0.91. Moreover, Hawkins et al. analyzed the national lung cancer screening tests in the CT image data and revealed that omics characteristics from images can be used for benign and malignant lung nodules. For this model, its predictions showed that the AUC reached 0.75 (followed up for 2 years) and 0.83 (1 year follow-up), and this method was superior to the traditional image evaluation and CAD-aided analysis (33). In addition, radiomics analyses are also used to differentiate lung cancer from benign diseases, such as nodular granuloma and infectious nodules, in order to improve the diagnostic accuracy of pulmonary nodules (34-36). These studies further confirm the application value of radiomics analyses in the determination of pulmonary nodules' benign and malignant properties and also lay the foundation for the subsequent clinical analysis of the pulmonary nodules’ properties.
The SSN properties can also be analyzed accurately using image omics analyses. For example, Yuan et al. (37). extracted 300 imaging features from the CT images of 431 patients with lung adenocarcinoma presenting with SSN, and 20 features were further screened to distinguish AIS, minimally invasive adenocarcinoma (MIA), and invasive adenocarcinoma (IA), proving that the accuracy and predictive ability of the radiomics methods were significantly higher than those of traditional methods. Even in sub-centimeter SSNs, the image omics analysis improved the diagnosis of IA (38). At the same time, the combination of radiomics analysis and intraoperative freezing is shown to improve the accuracy of predicting the final pathological diagnosis (39,40). In our study (41), by analyzing the image omics features and using the prediction model established by the LASSO classifier, the AUC in the verification set reached 0.890.
In addition to the analysis of benign, malignant and invasive nodules, radiomics analysis also predicts occult lymph node metastasis via the CT images (42,43). In our previous study, our team further analyzed the value of radiomics for the prediction of N2 lymph node metastasis (44). This series of studies confirmed that CT radiomics provides a noninvasive and accurate comprehensive assessment of pulmonary tuberculous properties.
Research progress in the study of the prognosis assessment of early stage lung cancer
Significance of CT images in the prognosis assessment of early stage lung cancer
CT image feature analyses not only reflect the nature of the pulmonary nodules, but also provide important information regarding the prognosis assessment of early stage lung cancer. First of all, the nature of pulmonary nodules is an important prognostic feature. Compared with early stage lung cancer with pure solid lesions by CT, the long-term prognosis after complete resection of GGN lesions is excellent. In a study by Fu et al. (45), the 5-year relapse-free survival (RFS) for PGGN reached 100%, the 5-year RFS for PSN was 87.6%, and the 5-year RFS for pure solid lesions was 73.2%. At the same time, invasive evaluation features, such as consolidation-to-tumor ratio (CTR) and tumor disappearance ratio (TDR), can also be used to evaluate the prognosis of early stage lung cancer. For example, the overall survival (OS) at 5 years can reach 96.7% for non-invasive lesions when defined as lesions with a CTR ≤0.5. However, some studies point out that the prognostic value of CTR or TDR can be corrected by existing clinical T staging, and only clinical staging is a prognostic factor (46). Therefore, CT images provide important data for analyzing the prognosis of early stage lung cancer, but the prognostic assessment methods based on traditional imaging features are very limited or the prognostic assessment effect of traditional imaging features is very limited.
The application of CT radiomics in lung cancer prognosis assessment and treatment decision making
In terms of survival assessment, Huang et al. analyzed CT images from 282 patients with early non-small cell lung cancer (NSCLC) that were surgically resected, established a predictive model of radiomics features, and further established a radiomics nomogram for prognosis assessment in combination with clinical features. In the validation set, the predictive ability (C-index) of this model reached 0.72 and was shown to improve the predictive ability of TNM staging (47). At the same time, the team of Chinese Academy of Sciences Professor Jie Tian (48) further confirmed that both two-dimensional and three-dimensional radiomics features could be used to evaluate the prognosis of NSCLC, and a nomogram model was established to predict the prognosis, showing a better predictive ability than the clinical model in the validation set. For adenocarcinoma, Lee et al. (49) extracted 161 CT imaging features from 339 patients, and LASSO was used as the feature screening and model-building classifier. Ultimately, a nomogram model for prognosis prediction was established in combination with the clinical features, and the AUC was predicted to reach a maximum of 0.864 in the verification set.
Meanwhile, Coroller’s team not only demonstrated the value of radiomics for the prognosis assessment, but also provided a new auxiliary decision-making method for clinical neoadjuvant chemoradiotherapy. The team utilized 127 patients with locally advanced NSCLC (stage II or III) before and after neoadjuvant therapy. Using the CT images from the patients, 1,603 features are extracted, and the 15 most predictive value characteristics were screened and compared with three traditional image features, demonstrating that predicting lesions after concurrent chemoradiation image group characteristics significantly alleviated the pathological efficiency and further showed that the seven characteristics effectively predicted the gross residual disease [gross residual diseases (GRD)]. Importantly, one feature effectively predicted the pathological complete response (pCR) (50). Again at the same time, the team utilized the CT images from 85 patients with resectable stage II and III NSCLC. The extraction of 85 and 178 lymph nodes from the primary lesion of 10 each image set of characteristics predicted an AUC pCR that reached 0.51 to 0.75, and a GRD AUC that reached 0.50 to 0.67. Compared with the characteristics from the primary lesion, the lymph node is characteristic of its source and has a higher predictive value. In addition, the team analyzed of 187 patients with lung adenocarcinoma that had preoperative CT images and extracted 635 features. They sorted out the 35 images with distant metastasis 12 for survival using the predictive omics characteristics and set up an independent predicting distant metastasis model of lung adenocarcinoma patients that was carried out in accordance with the transfer of high and low risk.
In addition, by analyzing the CT images of 117 stage IV NSCLC patients with the EGFR mutation, Song et al. (51) extracted 1,032 imaging group features and selected 12 features using the LASSO regression model to establish markers that would benefit from EGFR-TKIS, which effectively screened out patients who benefited from the treatment and assisted in the decision-making for the clinical treatment. Moreover, to evaluate immunotherapy, Sun et al. (52) established a model to predict CD8 expression, which predicted an AUC of 0.67 and was used to identify patients with different types of immune responses, with an AUC of 0.76, ultimately providing a non-invasive assessment tool for immune efficacy. Meanwhile, Trebeschi et al. (53). analyzed 1,055 primary or metastatic lesions of 203 melanoma or NSCLC cases, and image markers were established to predict the immune efficacy by machine learning. For the lung cancer patients, the predictive AUC of this marker reached 0.83, and for the melanoma patients, the predicted AUC of this marker reached 0.64. In addition, Khorrami et al. (54) retrospectively analyzed CT images from 50 NSCLC patients. They screened 8 radiomics features and established the radiomics score known as DelRADx. In two independent validation sets, DelRADx showed predicted AUC values of 0.81 and 0.85. At the same time, DelRADx was associated with lymphocyte density in tumor invasion in 35 diagnosed specimens. Finally, DelRADx was shown to predict the OS of the patients receiving treatment, with a C-index of 0.72. Our team further investigated the correlation between DL radiomic biomarker and tumor mutational burden. By combining DL technology and CT images, we developed an individual non-invasive biomarker that could distinguish high-tumor mutational burden from low-tumor mutational burden, which might inform decisions on the use of immune checkpoint inhibitors in patients with advanced NSCLC (55).
Therefore, CT radiomics analyses are widely used in the prognosis assessment of lung cancer and show a great clinical application value. Image analyses can accurately assess the prognosis of patients and predict tumor recurrence, thus assisting clinicians to utilize targeted treatments in order to reduce the risk of recurrence and improve outcome. At the same time, radiomics analyses can be used to assist decision making for adjuvant treatment.
Challenges of CT radiomics analyses in early stage lung cancer
Although radiomics has shown great potential in CT image analyses, traditional analysis methods still have the following problems: (I) the value of radiomics in lung cancer patient care was still at research stage; (II) a large number of image omics features are extracted, and there is instability, and the application features of each model are not uniform; and (III) the features require a dimensionality reduction, and there is no unified model selection standard. Specifically, in the above studies, the radiomics feature extraction of lung cancer is related to its segmentation, while for the SSN class of lesions, due to its low density characteristics, the boundary is not easy to determine, and the error range of the volume and mass segmentation changes by about 30% (56). At the same time, in addition to the imaging features of the lesions themselves, recent studies indicated that the peripheral imaging features of the lesions reflect the characteristics of the tumor micro-environment and also play an important role in the diagnosis and prognosis of the pulmonary nodules (34). Specifically, when evaluating immunotherapy patients (52), the quesiton of how to determine the region of intertest (ROI) in the tumor images and obtain accurate features is controversial. There are a large number of radiomics features that can be extracted from chest CT images. During the establishment of the model, artifical intellengence (AI) technology is required to repeatedly verify its accuracy, but there is no uniform standard. In addition, how to efficiently diagnose pulmonary nodules in CT images is a difficult clinical dilemma for traditional radiomics analyses (57). Such studies still have the following limitations: (I) the use of lung cancer screening test data showed regional bias; (II) fail to conduct adequate clinical comparison and practical application value assessment; (III) less assessment of the survival and prognosis of patients was carried out. The above problems have caused great resistance to CT radiomics analyses in the early stage of lung cancer (58). After all, there are much more challenges than the ones mentioned. A lot of research and validation still needs to be performed.
DL and CNN
Recently, with the leap in the development of computer hardware and the arrival of the era of big data, DL has become the most potential radiomics analysis technology. The working principle of DL is the artificial neural network (ANN) (59). The structure of ANN includes the input layer, hidden layer, and output layer, among which the hidden layer can be increased and decreased according to the different purposes. Thus, DL can be trained end-to-end by representing more and more abstract concepts level by level. In contrast, the DL algorithm can be used to extract a large number of high-dimensional features in a fully automated manner, enabling researchers to extract and utilize features of predictive value with minimal labor. DL has been successfully applied to computer vision, speech recognition, natural language processing, machine translation, data mining, automatic driving, and other fields (60).
CNN
According to the different DL network structures, they can be divided into CNN, recursive neural network, cyclic neural network, deep neural network, etc. Among these, CNN processes each specified pixel area in the image to enhance the information continuity of the image (61). At the same time, CNN has the feature of weight sharing, such a network structure cannot only significantly reduce the complexity of the model, but it also reduces the number of weights (62). In addition, the CNN can directly take the image as the input of the network, automatically extract the image features, and automatically train and adjust the model results (63). Therefore, CNN advocates a strong image data processing capability in the field of image recognition. With regard to the basic ANN, the hidden layer of CNN can be divided into the convolution layer and the pooling layer (64). The convolutional layer extracts features through the translation of the convolution kernel on the original image. The pooling layer is used to select the features, reduce the number of features, compress the number of data and parameters, reduce the over-fitting, and improve the fault tolerance of the model (15).
Several classical CNNs
In order to promote the development of the CNN technology, since 2007, Li Feifei, a professor of Stanford University, has led her team to establish an Image Net Image dataset using various methods, such as manual annotation, network capture, and a crowdsourcing platform (65). Based on this data set, an ImageNet Large-Scale Visual Recognition Challenge (ILSVRC) for CNN classification effects is held annually. The competition includes image classification, target positioning, target detection, video target detection, and scene classification (66).
Krizhevsky et al. (67) proposed the first modern deep CNN model, namely Alex Net, in 2012, which consists of 5 convolutional layers, 3 pooling layers, and 3 full connection layers (the last layer USES softmax function output). It first adopted many of the modern deep convolution network technology methods, such as using the image Processing Unit (Graphic Processing Unit, the GPU) running and training, and adopted the ReLU as a nonlinear activation function, using the Dropout prevention fitting, and it also used the data to enhance the accuracy of the models. Alex net won the 2012 ILSVRC localization and classification race champion.
Moreover, researchers in Oxford University’s Visual Geometry Group (VGG) and Google deep blue developed VGG Net together (67). By repeatedly adding a convolutional layer and a classification layer, VGG Net successfully constructed a CNN model with a depth of 16–19 layers, and the model won 2nd place in the ILSVRC classification competition and 1st place in the positioning competition in 2014. The 1st place winner in the same year was Google’s Inception Net. The network is 22 layers deep and has only 5 million parameters, which is only 1/12 of the number of Alex Net (60 million). However, the accuracy rate is much higher than Alex Net. Inception v2-v4 was launched by the Google team in 2015–2016.
In the design of CNNs, as the number of network layers increases, more deep features can be provided. However, as the network layer continues to increase, it will generate a fitting. Such problems are solved by a regularization method, but this also causes network degradation, namely the network model training data accuracy, after saturation, begins to decline. To this end, a team from Microsoft Research proposed to solve the problem by using a residual unit (RU). Based on the unit’s design of the 152-story ResNet (68), the team won the classification and positioning competitions at the ILSVRC in 2015.
Advantages of DL artificial intelligence technology
DL neural networks can process more data to improve their prediction abilities. Traditional AI algorithms usually achieve a certain amount of data, while the prediction accuracy will not continue to improve with the increased data volume. For example, with increased image data, CNN captures deeper features to improve the accuracy of the classification (69). Therefore, for a massive dataset of chest CT images, the accuracy can approach the level of clinicians after training the CNN model. This enabled us to hand over the tedious and repeated clinical work to the machine for processing. Combined with the review of clinicians, the pre-processing of chest CT images and the detection of pulmonary nodules can be completed efficiently (70).
What is more important, the DL neural network does not require complex feature engineering, such as the pre-defined feature extraction in the traditional image grouping analysis. In the DL neural network, end-to-end model training can be used to directly take the image as the input and automatically adjust the parameters to obtain the best prediction results. Therefore, DL neural networks can solve the process of image segmentation and feature screening in traditional radiomics analyses (71). It also can include the information reflecting the characteristics of the tumor microenvironment into the analysis according to the expansion of the input image, so as to improve the accuracy of the CT image analysis of the nature of early stage lung cancer (72).
In addition, DL neural networks have a strong adaptability and are easy to transform. They can adapt to different fields and applications more easily. For example, transfer learning enables pre-trained DL neural networks to be adapted for different purposes in different fields. In the study by Kermany et al. (73), after pre-training a dataset of up to 1,000 image classifications in Image Net, the model can be transferred to the diagnosis of clinical diseases, so as to achieve an accurate classification of diabetic retinopathy and pediatric pneumonia. Furthermore, the basic techniques and ideas of DL used are often convertible even in different domains, such as speech recognition, where the baseline knowledge of the DL theory is highly similar to that of the basic DL theory in natural language processing.
Conclusions
To sum up, accurate assessment of the nature of early stage lung cancer and prognosis of patients is currently the focus of thoracic surgery research. From Mayo model to the current CT image omics, early lung CT image analysis has experienced the process from quality to quantity (Figure 1). Although the traditional radiomics has shown a strong analysis ability, its limitations in practical application have been gradually highlighted. CNN, as a new radiomics analysis technology, has been applied to the CT image study of early stage lung cancer. We hope that many promising and potential multidisciplinary researches on functional imaging could be carried out to raise the clinical applications as well as to increase benefits of NSCLC patients.
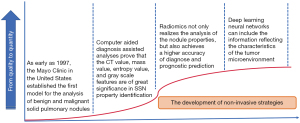
Acknowledgments
Funding: Supported by the projects from National Natural Science Foundation of China (grant number 91959126), Public Health Scientific Projects in Zhejiang Province (2020KY270), and Huamei Key Research Foundation (2019HMZD05), Shanghai Pulmonary Hospital Program (grant number fkcx1906), and Shanghai Yangfan Program (grant number 20YF1441100).
Footnote
Provenance and Peer Review: This article was commissioned by the Guest Editors (Wieland Voigt and Helmut Prosch) for the series “Lung Cancer Screening” published in Shanghai Chest. The article has undergone external peer review.
Reporting Checklist: The authors have completed the Narrative Review Checklist. Available at: http://dx.doi.org/10.21037/shc-20-81
Peer Review File: Available at http://dx.doi.org/10.21037/shc-20-81
Conflicts of Interest: The authors have completed the ICMJE uniform disclosure form (available at http://dx.doi.org/10.21037/shc-20-81). The series “Lung Cancer Screening” was commissioned by the editorial office without any funding or sponsorship. The authors have no other conflicts of interest to declare.
Ethical Statement: The authors are accountable for all aspects of the work in ensuring that questions related to the accuracy or integrity of any part of the work are appropriately investigated and resolved.
Open Access Statement: This is an Open Access article distributed in accordance with the Creative Commons Attribution-NonCommercial-NoDerivs 4.0 International License (CC BY-NC-ND 4.0), which permits the non-commercial replication and distribution of the article with the strict proviso that no changes or edits are made and the original work is properly cited (including links to both the formal publication through the relevant DOI and the license). See: https://creativecommons.org/licenses/by-nc-nd/4.0/.
References
- Izumchenko E, Chang X, Brait M, et al. Targeted sequencing reveals clonal genetic changes in the progression of early lung neoplasms and paired circulating DNA. Nat Commun 2015;6:8258. [Crossref] [PubMed]
- Horeweg N, Scholten ET, de Jong PA, et al. Detection of lung cancer through low-dose CT screening (NELSON): a prespecified analysis of screening test performance and interval cancers. Lancet Oncol 2014;15:1342-50. [Crossref] [PubMed]
- Aberle DR, Adams AM, Berg CD, et al. Reduced lung-cancer mortality with low-dose computed tomographic screening. N Engl J Med 2011;365:395-409. [Crossref] [PubMed]
- National Lung Screening Trial Research Team. Reduced Lung-Cancer Mortality with Low-Dose Computed Tomographic Screening. 2011;365:395-409.
- de Koning HJ, van der Aalst CM, de Jong PA, et al. Reduced Lung-Cancer Mortality with Volume CT Screening in a Randomized Trial. N Engl J Med 2020;382:503-13. [Crossref] [PubMed]
- Swensen SJ, Silverstein MD, Ilstrup DM, et al. The probability of malignancy in solitary pulmonary nodules. Application to small radiologically indeterminate nodules. Arch Intern Med 1997;157:849-55. [Crossref] [PubMed]
- Lee G, Lee HY, Park H, et al. Radiomics and its emerging role in lung cancer research, imaging biomarkers and clinical management: State of the art. Eur J Radiol 2017;86:297-307. [Crossref] [PubMed]
- Aerts HJ. The Potential of Radiomic-Based Phenotyping in Precision Medicine: A Review. JAMA Oncol 2016;2:1636-42. [Crossref] [PubMed]
- Lambin P, Leijenaar RTH, Deist TM, et al. Radiomics: the bridge between medical imaging and personalized medicine. Nat Rev Clin Oncol 2017;14:749-62. [Crossref] [PubMed]
- Verma V, Simone CB 2nd, Krishnan S, et al. The Rise of Radiomics and Implications for Oncologic Management. J Natl Cancer Inst 2017;109. [Crossref] [PubMed]
- Raghunath S, Maldonado F, Rajagopalan S, et al. Noninvasive risk stratification of lung adenocarcinoma using quantitative computed tomography. J Thorac Oncol 2014;9:1698-703. [Crossref] [PubMed]
- Song SH, Park H, Lee G, et al. Imaging Phenotyping Using Radiomics to Predict Micropapillary Pattern within Lung Adenocarcinoma. J Thorac Oncol 2017;12:624-32. [Crossref] [PubMed]
- Rios Velazquez E, Parmar C, Liu Y, et al. Somatic Mutations Drive Distinct Imaging Phenotypes in Lung Cancer. Cancer Res 2017;77:3922-30. [Crossref] [PubMed]
- Chartrand G, Cheng PM, Vorontsov E, et al. Deep Learning: A Primer for Radiologists. Radiographics 2017;37:2113-31. [Crossref] [PubMed]
- Hosny A, Parmar C, Quackenbush J, et al. Artificial intelligence in radiology. Nat Rev Cancer 2018;18:500-10. [Crossref] [PubMed]
- Bi WL, Hosny A, Schabath MB, et al. Artificial intelligence in cancer imaging: Clinical challenges and applications. CA Cancer J Clin 2019;69:127-57. [Crossref] [PubMed]
- MacMahon H, Austin JH, Gamsu G, et al. Guidelines for management of small pulmonary nodules detected on CT scans: a statement from the Fleischner Society. Radiology 2005;237:395-400. [Crossref] [PubMed]
- Herder GJ, van Tinteren H, Golding RP, et al. Clinical prediction model to characterize pulmonary nodules: validation and added value of 18F-fluorodeoxyglucose positron emission tomography. Chest 2005;128:2490-6. [Crossref] [PubMed]
- Gould MK, Ananth L, Barnett PG. A clinical model to estimate the pretest probability of lung cancer in patients with solitary pulmonary nodules. Chest 2007;131:383-8. [Crossref] [PubMed]
- Schultz EM, Sanders GD, Trotter PR, et al. Validation of two models to estimate the probability of malignancy in patients with solitary pulmonary nodules. Thorax 2008;63:335-41. [Crossref] [PubMed]
- Yonemori K, Tateishi U, Uno H, et al. Development and validation of diagnostic prediction model for solitary pulmonary nodules. Respirology 2007;12:856-62. [Crossref] [PubMed]
- Li Y, Chen KZ, Wang J. Development and validation of a clinical prediction model to estimate the probability of malignancy in solitary pulmonary nodules in Chinese people. Clin Lung Cancer 2011;12:313-9. [Crossref] [PubMed]
- Al-Ameri A, Malhotra P, Thygesen H, et al. Risk of malignancy in pulmonary nodules: A validation study of four prediction models. Lung Cancer 2015;89:27-30. [Crossref] [PubMed]
- She Y, Zhao L, Dai C, et al. Development and validation of a nomogram to estimate the pretest probability of cancer in Chinese patients with solid solitary pulmonary nodules: A multi-institutional study. J Surg Oncol 2017;116:756-62. [Crossref] [PubMed]
- McWilliams A, Tammemagi MC, Mayo JR, et al. Probability of cancer in pulmonary nodules detected on first screening CT. N Engl J Med 2013;369:910-9. [Crossref] [PubMed]
- Tammemagi MC, Mayo JR, Lam S. Cancer in pulmonary nodules detected on first screening CT. N Engl J Med 2013;369:2060-1. [Crossref] [PubMed]
- Zheng B, Zhou X, Chen J, et al. A Modified Model for Preoperatively Predicting Malignancy of Solitary Pulmonary Nodules: An Asia Cohort Study. Ann Thorac Surg 2015;100:288-94. [Crossref] [PubMed]
- She Y, Zhao L, Dai C, et al. Preoperative nomogram for identifying invasive pulmonary adenocarcinoma in patients with pure ground-glass nodule: A multi-institutional study. Oncotarget 2017;8:17229-38. [Crossref] [PubMed]
- Lambin P, Rios-Velazquez E, Leijenaar R, et al. Radiomics: extracting more information from medical images using advanced feature analysis. Eur J Cancer 2012;48:441-6. [Crossref] [PubMed]
- Thawani R, McLane M, Beig N, et al. Radiomics and radiogenomics in lung cancer: A review for the clinician. Lung Cancer 2018;115:34-41. [Crossref] [PubMed]
- Wilson R, Devaraj A. Radiomics of pulmonary nodules and lung cancer. Transl Lung Cancer Res 2017;6:86-91. [Crossref] [PubMed]
- Wu H, Sun T, Wang J, et al. Combination of radiological and gray level co-occurrence matrix textural features used to distinguish solitary pulmonary nodules by computed tomography. J Digit Imaging 2013;26:797-802. [Crossref] [PubMed]
- Hawkins S, Wang H, Liu Y, et al. Predicting Malignant Nodules from Screening CT Scans. J Thorac Oncol 2016;11:2120-8. [Crossref] [PubMed]
- Beig N, Khorrami M, Alilou M, et al. Perinodular and Intranodular Radiomic Features on Lung CT Images Distinguish Adenocarcinomas from Granulomas. Radiology 2019;290:783-92. [Crossref] [PubMed]
- Yang X, He J, Wang J, et al. CT-based radiomics signature for differentiating solitary granulomatous nodules from solid lung adenocarcinoma. Lung Cancer 2018;125:109-14. [Crossref] [PubMed]
- Uthoff J, Nagpal P, Sanchez R, et al. Differentiation of non-small cell lung cancer and histoplasmosis pulmonary nodules: insights from radiomics model performance compared with clinician observers. Transl Lung Cancer Res 2019;8:979-88. [Crossref] [PubMed]
- Yuan M, Zhang YD, Pu XH, et al. Comparison of a radiomic biomarker with volumetric analysis for decoding tumour phenotypes of lung adenocarcinoma with different disease-specific survival. Eur Radiol 2017;27:4857-65. [Crossref] [PubMed]
- Zhao W, Xu Y, Yang Z, et al. Development and validation of a radiomics nomogram for identifying invasiveness of pulmonary adenocarcinomas appearing as subcentimeter ground-glass opacity nodules. Eur J Radiol 2019;112:161-8. [Crossref] [PubMed]
- Wang B, Tang Y, Chen Y, et al. Joint use of the radiomics method and frozen sections should be considered in the prediction of the final classification of peripheral lung adenocarcinoma manifesting as ground-glass nodules. Lung Cancer 2020;139:103-10. [Crossref] [PubMed]
- Wu G, Woodruff HC, Sanduleanu S, et al. Preoperative CT-based radiomics combined with intraoperative frozen section is predictive of invasive adenocarcinoma in pulmonary nodules: a multicenter study. Eur Radiol 2020;30:2680-91. [Crossref] [PubMed]
- She Y, Zhang L, Zhu H, et al. The predictive value of CT-based radiomics in differentiating indolent from invasive lung adenocarcinoma in patients with pulmonary nodules. Eur Radiol 2018;28:5121-8. [Crossref] [PubMed]
- Yang X, Pan X, Liu H, et al. A new approach to predict lymph node metastasis in solid lung adenocarcinoma: a radiomics nomogram. J Thorac Dis 2018;10:S807-19. [Crossref] [PubMed]
- Wang X, Zhao X, Li Q, et al. Can peritumoral radiomics increase the efficiency of the prediction for lymph node metastasis in clinical stage T1 lung adenocarcinoma on CT? Eur Radiol 2019;29:6049-58. [Crossref] [PubMed]
- Yang M, She Y, Deng J, et al. CT-based radiomics signature for the stratification of N2 disease risk in clinical stage I lung adenocarcinoma. Transl Lung Cancer Res 2019;8:876-85. [Crossref] [PubMed]
- Fu F, Zhang Y, Wen Z, et al. Distinct Prognostic Factors in Patients with Stage I Non-Small Cell Lung Cancer with Radiologic Part-Solid or Solid Lesions. J Thorac Oncol 2019;14:2133-42. [Crossref] [PubMed]
- Kim H, Goo JM, Kim YT, et al. Consolidation-to-tumor ratio and tumor disappearance ratio are not independent prognostic factors for the patients with resected lung adenocarcinomas. Lung Cancer 2019;137:123-8. [Crossref] [PubMed]
- Huang Y, Liu Z, He L, et al. Radiomics Signature: A Potential Biomarker for the Prediction of Disease-Free Survival in Early-Stage (I or II) Non-Small Cell Lung Cancer. Radiology 2016;281:947-57. [Crossref] [PubMed]
- Yang L, Yang J, Zhou X, et al. Development of a radiomics nomogram based on the 2D and 3D CT features to predict the survival of non-small cell lung cancer patients. Eur Radiol 2019;29:2196-206. [Crossref] [PubMed]
- Lee G, Park H, Sohn I, et al. Comprehensive Computed Tomography Radiomics Analysis of Lung Adenocarcinoma for Prognostication. Oncologist 2018;23:806-13. [Crossref] [PubMed]
- Coroller TP, Agrawal V, Narayan V, et al. Radiomic phenotype features predict pathological response in non-small cell lung cancer. Radiother Oncol 2016;119:480-6. [Crossref] [PubMed]
- Song J, Shi J, Dong D, et al. A New Approach to Predict Progression-free Survival in Stage IV EGFR-mutant NSCLC Patients with EGFR-TKI Therapy. Clin Cancer Res 2018;24:3583-92. [Crossref] [PubMed]
- Sun R, Limkin EJ, Vakalopoulou M, et al. A radiomics approach to assess tumour-infiltrating CD8 cells and response to anti-PD-1 or anti-PD-L1 immunotherapy: an imaging biomarker, retrospective multicohort study. Lancet Oncol 2018;19:1180-91. [Crossref] [PubMed]
- Trebeschi S, Drago SG, Birkbak NJ, et al. Predicting response to cancer immunotherapy using noninvasive radiomic biomarkers. Ann Oncol 2019;30:998-1004. [Crossref] [PubMed]
- Khorrami M, Prasanna P, Gupta A, et al. Changes in CT Radiomic Features Associated with Lymphocyte Distribution Predict Overall Survival and Response to Immunotherapy in Non-Small Cell Lung Cancer. Cancer Immunol Res 2020;8:108-19. [Crossref] [PubMed]
- He B, Dong D, She Y, et al. Predicting response to immunotherapy in advanced non-small-cell lung cancer using tumor mutational burden radiomic biomarker. J Immunother Cancer 2020;8:e000550 [Crossref] [PubMed]
- de Hoop B, Gietema H, van de Vorst S, et al. Pulmonary ground-glass nodules: increase in mass as an early indicator of growth. Radiology 2010;255:199-206. [Crossref] [PubMed]
- Choe J, Lee SM, Do KH, et al. Deep Learning-based Image Conversion of CT Reconstruction Kernels Improves Radiomics Reproducibility for Pulmonary Nodules or Masses. Radiology 2019;292:365-73. [Crossref] [PubMed]
- Wu W, He Z, Xu J, et al. Anatomical pulmonary sublobar resection based on subsegment. Ann Thorac Surg 2021;111:e447-50. [Crossref] [PubMed]
- Ham YG, Kim JH, Luo JJ. Deep learning for multi-year ENSO forecasts. Nature 2019;573:568-72. [Crossref] [PubMed]
- Skrede OJ, De Raedt S, Kleppe A, et al. Deep learning for prediction of colorectal cancer outcome: a discovery and validation study. Lancet 2020;395:350-60. [Crossref] [PubMed]
- Perl RM, Grimmer R, Hepp T, et al. Can a Novel Deep Neural Network Improve the Computer-Aided Detection of Solid Pulmonary Nodules and the Rate of False-Positive Findings in Comparison to an Established Machine Learning Computer-Aided Detection? Invest Radiol 2021;56:103-8. [Crossref] [PubMed]
- Su Y, Li D, Chen X. Lung Nodule Detection based on Faster R-CNN Framework. Comput Methods Programs Biomed 2021;200:105866 [Crossref] [PubMed]
- Coudray N, Ocampo PS, Sakellaropoulos T, et al. Classification and mutation prediction from non-small cell lung cancer histopathology images using deep learning. Nat Med 2018;24:1559-67. [Crossref] [PubMed]
- Gao R, Tang Y, Xu K, et al. Time-distanced gates in long short-term memory networks. Med Image Anal 2020;65:101785 [Crossref] [PubMed]
- Hofmanninger J, Prayer F, Pan J, et al. Automatic lung segmentation in routine imaging is primarily a data diversity problem, not a methodology problem. Eur Radiol Exp 2020;4:50. [Crossref] [PubMed]
- Lin C, Zhao G, Yang Z, et al. CIR-Net: Automatic Classification of Human Chromosome based on Inception-ResNet Architecture. IEEE/ACM Trans Comput Biol Bioinform 2020. doi:
10.1109/TCBB.2020.3003445 .10.1109/TCBB.2020.3003445 - Krizhevsky A, Sutskever I, Hinton GE. ImageNet Classification with Deep Convolutional Neural Networks. Adv Neural Inf Process Syst 2012;25:1097-105.
- Xie Y, Xia Y, Zhang J, et al. Knowledge-based Collaborative Deep Learning for Benign-Malignant Lung Nodule Classification on Chest CT. IEEE Trans Med Imaging 2019;38:991-1004. [Crossref] [PubMed]
- Wang S, Wang R, Zhang S, et al. 3D convolutional neural network for differentiating pre-invasive lesions from invasive adenocarcinomas appearing as ground-glass nodules with diameters ≤3 cm using HRCT. Quant Imaging Med Surg 2018;8:491-9. [Crossref] [PubMed]
- Wang Q, Shen F, Shen L, et al. Lung Nodule Detection in CT Images Using a Raw Patch-Based Convolutional Neural Network. J Digit Imaging 2019;32:971-9. [Crossref] [PubMed]
- Wang S, Zhou M, Liu Z, et al. Central focused convolutional neural networks: Developing a data-driven model for lung nodule segmentation. Med Image Anal 2017;40:172-83. [Crossref] [PubMed]
- Tu X, Xie M, Gao J, et al. Automatic Categorization and Scoring of Solid, Part-Solid and Non-Solid Pulmonary Nodules in CT Images with Convolutional Neural Network. Sci Rep 2017;7:8533. [Crossref] [PubMed]
- Kermany DS, Goldbaum M, Cai W, et al. Identifying Medical Diagnoses and Treatable Diseases by Image-Based Deep Learning. Cell 2018;172:1122-31.e9. [Crossref] [PubMed]
Cite this article as: Yang M, She Y, Ren Y, Deng J, Xie D, Sun X, Shi J, Chen C. Early-stage lung cancer detection from radiomics to deep learning in thoracic CT images: a narrative review with contemporary clinical recommendations. Shanghai Chest 2021;5:36.