Computed tomography lung-cancer screening: does iterative reconstruction matter?
Introduction
In the United States, lung cancer disease causes more deaths than other cancer type (1). The Lung Cancer Screening Trial NLST showed a possible relative risk reduction of dying from lung cancer in the risk group by 20% by performed repeated low-dose computed tomography (LDCT) screening procedures, which corresponds to an absolute risk reduction of 0.3% (2,3). The Belgian-Dutch randomized-controlled NELSON Trial demonstrated a reduction of lung cancer mortality with LDCT for high-risk patients over a 10-year period: men by 26% and women between 39% and 61% (4,5). These results have provided sufficient evidence for the European Union to draft a policy statement on lung cancer screening (LCS) using LDCT (5,6). The majority of detected cancer cases found in the NLST and NELSON screening studies were stage I cancers (2,7-10). This shows the ability of LDCT to detect lung cancer early and so potentially enable cure.
The use of LDCT for LCS has inherent risks, which were identified in the different trials and the following evaluation processes (11). First, the computed tomography (CT) associated risk of radiation induced morbidity, which not only derives from the repeated LDCT scans, but especially from the diagnostic work-up of incidental findings. According to (12) the necessary diagnostic radiation exposure would cause one radiation-induced major cancer for every 108 (259/2.4) lung cancers detected through screening. Second, risks come from adverse events during diagnostic procedures after screening, overdiagnosis and negative effects of screening on smoking cessation (13). There are various approaches in discussion to overcome these risks. Among others the optimal screening interval is important for harm minimization (14). But the reduction of radiation dose by maintaining a sufficient diagnostic performance will be paramount for the acceptance of screening programs and implementing LCS in general population.
A recently published systematic review and meta-analysis of randomized controlled trials of LCS with LDCT in (former) smokers revealed a significant reduction (12%) of lung cancer mortality and estimated the risk of overdiagnosis between 19% and 69% (15). As of March 2021, the U.S. Preventive Services Task Force recommends annual screening for lung cancer with LDCT in adults aged 50 to 80 years who have a 20 pack-year smoking history and currently smoke or have quit within the past 15 years (16).
Since its introduction in 1970’s, CT technology has undergone numerous developments, from single-section CT scanners to multi-detector systems that can acquire entire chest in only few seconds. Technology progresses in X-ray tubes, e.g., flying focus, shortage in rotation time, enlargement of detector size, advanced reconstruction algorithms had become available. To meet high quality standards in terms of dose reduction and image quality, manufacturers created multiple solutions, e.g., reduction of tube voltage or automatic tube current modulation (17). Dose reduction, however, causes noisy images, which can be partly improved by using vendor’s dose-management LDCT protocols.
The NELSON and the NLST investigations are based on repeated CT examinations with low-dose protocols and filtered back projection (FBP) reconstruction (1,4-6). Although high contrast LDCT protocols can be applied for lung foci detection, a disadvantage is the usage of the historically evolved FBP. Iterative reconstruction (IR), specifically model-based IR, offers a promising alternative and complement with the potential of higher image fidelity. An advantage of IR is its inherent discrete formulation which allows to model the complete CT acquisition process, including detector geometry and sensitivity, beam shape, physics of the X-ray quanta as well as the use of prior knowledge like electronic noise. Statistical IR reconstruction may incorporate statistics of detected photons and can be used in the raw, in the image data domain or during the reconstruction process. Model-based IR even extends beyond by embedding geometric and physical models of the components of the CT scanner (18).
The effective dose and convolution kernels effects on the detection of pulmonary nodules in IR were investigated in studies with anthropomorphic lung phantoms by quantifying the perception of expert radiologists’ at a Likert scale, contrast-to-noise and signal-to-noise ratio (SNR) (19-21). Other studies evaluated machine learning (ML), computer-aided detection (CAD) software systems or commercially available deep learning ML based CAD systems by investigating receiver-operating-curve measures in artificial or ex-vivo lung phantoms (22-25). In the future ML algorithms might enable risk assessment to determine the indication for lung scans in clinical routine and even define CT imaging parameters. Beside the detection of pulmonary nodules, diagnostic applications may include automatic lesion segmentation or quantification of parenchymal changes like fibrosis or emphysema.
The aim of this work is twofold. Firstly, based on a short review of principles of CT and FBP reconstruction compared to IR—as far as possible in non-mathematical terms—we will, secondly, present CT phantom studies for the pulmonary nodule detection using FBP and IR to address the interdependence between IR strength levels, kernels and LDCT protocols.
Methods
The system hardware of a radiological CT is divided into the scanner equipment and the display or operating hardware (26). The scanner design of standard CT systems used clinically is assigned to the development stage of the third CT generation, shown in Figure 1. The technical realisation of this development stage amounts to three main components. The X-ray tube (source) for radiation generation with the coupled measuring unit (detector), as well as the positioning device (table). The source-detector combination is carried by the CT gantry. During the CT experiment, the recording geometry rotates on a defined circular path around the isocentre in the measurement field.
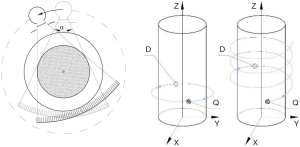
A specific feature of the third CT generation represents a source with an upstream collimator, through whose aperture in the axial plane a fan beam with an aperture angle between 40° and 60° is generated. Spiral mode has revolutionized CT imaging (24), the table is continuously advanced during the measurement forming a helical trajectory. Schematic representations of the circular and spiral trajectories are shown in Figure 1.
Modern X-ray tubes have a periodically z-flying focal spot (27,28). In fan-beam or single-line CT, the detector array consists of a straight or curved detector row (line) of several individual detector elements (26). State-of-the-art CT systems contain multi-raw detectors providing multi-slice images. Here, the collimator system on the source side is additionally opened in the z-direction and a cone beam geometry is generated.
CT system variants with two source-detector combinations implemented as dual-source CT exist for the third CT generation (29). Future CT systems will, among others, include photon counting detectors enabling energy resolved CT scanning, low-kV scanning, and sparse acquisition (30).
The result of the CT scanning are projections, i.e., logarithm of detected and normalized intensity, called sinogram. The fundamental task of CT reconstruction is to solve an inverse problem, i.e., calculate the attenuation coefficients from acquired projections. FBP is an analytical reconstruction method widely implemented on state-of the art CT scanners. The attenuation coefficient is obtained by back projecting filtered sinogram using a dedicated filter suitable for the anatomy under investigation (18). An edge-emphasising filter is called hard, and this applies analogously to associated convolution kernels. Filters and kernels that have strong smoothing properties are called soft.
Today’s IR is based on a statistical approach. The number of detected quanta is assumed to be Poisson distributed. The extension towards model-based IR considers the specific scanner geometry and its components, i.e., the entire process of acquisition. Also, prior knowledge about the anatomy may be implemented in the reconstruction for the purpose of noise reduction (18).
In this work, the Advanced Modeled Iterative Reconstruction (ADMIRE), a Siemens Healthineers (15) implementation of a model-based IR was used (18). It comprises two loops schematically shown in Figure 2. Loop A works across the raw and image data space and mainly removes spiral artefacts. Loop A starts from the initial image volume with the forward projection to calculate the pseudo-sinogram. Loop B corrects for noise and optimises the estimated image in image space by constraining an oversmoothed image texture. Loop B optimises the image step by step using the noise model and the regularisation function until an a-priori termination criterion is met and the reconstruction is completed with the final image volume.
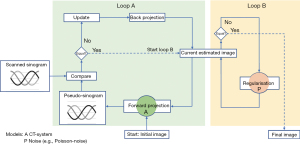
ADMIRE offers image-noise control at five strengths levels, S1–S5. The number of iterations in the raw data space as well as the calculation speed are not influenced by the choice of the strength. The recommended noise strength is S3. Finally, the operator preselects the reconstruction kernel (17). ADMIRE reconstruction works efficiently with low numbers of detected X-ray photons and low signal levels, it reduces patient’s dose for a fixed CT dose protocol by up to 60% compared to FBP reconstruction while providing comparable image quality (19).
In general, dose reduction approaches are subject to a broad spectrum. There are software and hardware approaches by the manufacturers, which can be supplemented by radiation protection measures on the part of the user. The latter refer to patient preparation, centring, and positioning aspects. Particularly, exact patient centring in the isocentre and an efficient use of tube-side-form filters prevents image quality losses. Overhead positioning of the arms during CT of the thorax will decrease photo absorption in the examination area. This may include the avoidance of metallic objects during active tube current modulation. Scan length reduction, selection of appropriate scan parameters, combined imaging methods, the use of radiation protection agents and the saving of partial examinations by dual-energy CT techniques are other measures to manage dose. For example, one CT scanner vendor modulates the tube voltage based on the topogram, specifically, patient diameter profile, system performance and the selected CT dose protocol (17). Other vendors offer similar dose management.
In this investigation, raw data acquisitions of phantoms placed supine head-first were acquired on a Somatom Force CT equipped with a VectronTM X-ray tube and a StellarInfinity detector with anti-scatter 3D collimator grid (17). The detector acquisition configuration was 192×0.6 mm and the z-flying focal spot technique was enabled. Three dose protocols were selected: (I) standard high contrast (SH) 120 kV/dose modulation, (II) low-dose 120 kV/40 mAs and (III) ultra-low-dose 120 kV/20 mAs. Phantoms were scanned using a field of view of 300 mm, rotation time 0.5 s, delay 2 s, beam collimation 192×0.6 mm, deactivated CAREDose4D, slice thickness 1 mm, increment 1 mm. Depending on the specific investigation the following IR kernels were used: Bl57 as standard for lung node detection, Br32 as a soft, and Br69 as a hard kernel. The images were reconstructed with the IR strength levels S1, S3–S5 and axial slices of 5 mm thickness, 5 mm reconstruction increment, and with parenchymal lung window (−600/1,200 HU) (17-19). HU denotes Hounsfield units.
CT images were reconstructed using FBP and IR algorithm ADMIRE and classified according to their suitability for lung node detection. To this end, statistical and subjective tests were conducted on CT images of artificial lung nodules in two anthropomorphic phantom bodies acquired using above mentioned dose protocols and kernels.
Pulmonary lesions modelled by artificial lung nodules were inserted to the phantoms. One nodules set is shaped as spheres with diameters 3/5/8/10/12 mm. Another nodule set is shaped as spicules with total diameters 16/20/24 mm. Both sets are manufactured, customized on purpose, and calibrated to −690/−50/+100 HU at 120 kV by QRM GmbH, Moehrendorf, Germany (31).
The anthropomorphic QRM phantom was equipped artificial lung nodules to simulate an adult human chest (Figure 3). It consists of calibrated Hounsfield values components representing tissue. The chest size was defined by extension rings, M (medium) and L (large), with effective height of 25/30 cm and width of 35/40 cm (31).
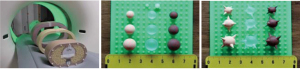
Statistical analysis
From a total number of 36 images four randomly selected images with IR strength S1, S3–S5 were randomly placed in a 4-field graphic interface. Sample criteria were: QRM phantom with ring L, variable kernels Bl57, Br32, Br69 and IR strength S1, S3–S5. To study body diameter, the second sample of images was varied to include Bl57 kernel reconstructions with ring L and M. Each sample contains 36 reconstructions, which were anonymised. The 4-field panel is presented to six expert radiologists asking to rank the images according to perception in a descending order. A Kappa and a modified Kappa test as well as Friedman values (F-values) were performed, and the later compared to a critical value, i.e., representing error probability at α=5% level (19-21).
The Lungman N1 phantom manufactured by Kyoto Kagaku, Kyoto, Japan, is a multipurpose life-size anatomical model that replicate an adult human chest (Figure 4) (32). It consists of components with calibrated Hounsfield values (HU) of human tissue: Chest wall with synthetic bones, pulmonary vasculature with mediastinum (trachea and heart) and diaphragm abdominal block. Tissue equivalent materials for the human habitus, inner components and nodes are made from polyurethane, urethane foam, epoxy resin and calcium carbonate. Previously mentioned artificial lung nodules are glued on positions selected by distinguished radiologists and are displayed in Figure 4.
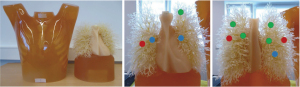
Results
Radiologist’s perception rankings of IR reconstructed QRM phantom images in the 4-field panel were averaged. Averaged rank sums over all test sets are displayed in Figure 5. The collective result shows that medium to high IR strength (≥ S3) were generally rated higher, S1 received lowest ranking. The assessment of the radiologist with 26 years of professional practice (ypp) does not follow the cumulative result, whereas the most (32 ypp) and less experienced observers (1 ypp) rated in accordance with the overall result.
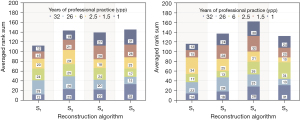
Ranking tests of iterative CT reconstructions show that radiologists consider medium to high IR strength more suitable for lung foci detection. If clinically applied parameters were used (Bl57, varying phantom diameter), the experiment reveals a noticeable preference of S4.
For the Lungman phantom eight medical technology engineers performed SNR measurements in the 72 test images to determine objective image quality parameters. One region of interest (ROI) was placed in the right lung (landmark RL) and one in the left lung (landmark LL), as shown in Figure 6.
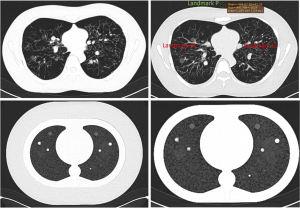
The mean SNR and the standard deviation were calculated from all measurements. The results have been sorted by geometry (sphere vs. spicule) and landmark LL vs. RL. This was followed by statistical analysis with a one-tailed t-test for paired samples. The results are displayed in Figure 7.
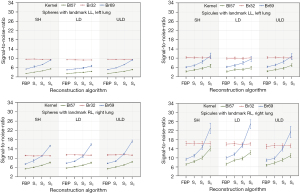
The subjective perception of the image quality was carried out by three expert radiologists with different previous professional experience. The 72 test series of the Lungman phantom were evaluated regarding the detectability of the artificial pulmonary nodules at a Likert scale. The six levels represented percentage ranges with assigned classification: 100%= excellent, 99–80%= good, 79–65%= moderate, 64–50%= adequate, 49–20%= poor and 19–0%=unacceptable.
Table 1 shows the number of radiologists’ individual maximum scores for each reconstruction algorithm and kernel. The ranking of the reconstruction method—regardless of kernels and nodule’s geometry—obtained by summing the maximum scores per line reveals FBP (with 33) on top followed by IR (with S3: 26, and S1: 25). The totals of the maximum scores per row—regardless of reconstruction method—demonstrate higher perception for spheres compared to spicules. Specifically, the Bl57 kernel outperforms Br69 and Br32 for spheres and spicules. These findings are consistent with the analysis of the number of radiologists’ individual minimum scores.
Table 1
Reconstruction/Kernel | Spheres | Spicules | Sum | |||||
---|---|---|---|---|---|---|---|---|
Bl57 | Br32 | Br69 | Bl57 | Br32 | Br69 | |||
FBP | 8 | 3 | 7 | 7 | 3 | 5 | 33 | |
IR/S1 | 5 | 4 | 5 | 5 | 2 | 5 | 25 | |
IR/S3 | 7 | 4 | 6 | 5 | 1 | 3 | 26 | |
IR/S5 | 5 | 1 | 3 | 2 | 4 | 2 | 17 | |
Sum | 24 | 12 | 21 | 19 | 10 | 15 |
FBP, filtered back projection; IR, iterative reconstruction.
Discussion
Ranking tests with the QRM phantom images suggest radiological preference of medium to high strength levels of the IR for lung foci detection. If clinically applied parameters were used (Bl57, varying phantom diameter), the experiment reveals a noticeable preference for S4. We hypothesise, that in the subjective image quality analysis the assessment habits of radiologists are dichotomous—or multiple stage—categorical. Despite differences in specialties and diagnostic habituation, most experienced and less experienced observers scored similarly. All observers have confidently selected rank positions 1 and 4, i.e., the best and the worst image. The intra-observer reliability for the rank positions 2 and 3 was significantly lower (20,21).
Lungman phantom images reconstructed with FBP were rated with highest perception by experienced radiologists. Less experienced readers assessed FBP and IR equally with the highest grades for IR with the Bl57 kernel. Scores for the IR depend on the dose protocol and kernel. The SNR is higher in low-dose and ultra-low-dose protocols with the BL57 kernel and high IR strength level. SNR differences between spheres and spicules could be substantiated by slightly different placement of spheres and spicules.
Due to the steepest increase on ML-based image interpretation studies in recent years, sometimes ML-approaches using CAD need to be distinguish from ML prototypes (24). A severe limitation to traditional CAD approaches is an inability to acquire knowledge from new information. Thus, various research groups investigate deep learning ML approaches (23-25). In a proof-of-concept study reported in (25), the potential of deep learning ML software system has been investigated with the conclusion that results strongly agree with expert radiologist determination of lung nodule detection. Diagnosis of lung nodules on a per-nodule basis is highly sensitive, but poorly specific, with false-positive rates like those of radiologists.
In this investigation we specifically focused on the qualitative and quantitative impact of the statistical model-based IR in various LDCT protocols being measured by expert radiologist in terms of perception and SNR. This study has been conducted with a limited number of readers and one single CT scanner. This limitation is due to our today’s availability of state-of-the-art scanners equipped with model-based IR and low-dose lung protocols and will be certainly lifted in the future. Image quality assessments with lung phantoms are favorable as the impact of breathing, which may decorrelate the results, is explicitly excluded. Although both anthropomorphic phantoms used in this work are accurately calibrated to lung tissue, patient images might reveal additional crucial aspects, calling for detailed and advanced investigations.
We have detected that the model-based IR is suitable for lung-nodule detection in phantoms at markedly decreased radiation exposure. The CT protocols in the NELSON and in the NLST trial are based on repeated CT examination with low-dose protocols without IR, since this was introduced in clinical routine after 2010. The impact of IR was also demonstrated in (33), who reported ultra-low-dose with model-based IR of 0.13 mSv. This ranges in the dose of a standard chest radiograph, demonstrating the future potential of IR-based CT for a wide implementation of LCS. Subjective image perception of expert radiologist does not significantly correlate with their experience, which presumably mirrors reader’s experience. In a SNR investigation, IR outperforms filtered-back projection for spheres and spiculated nodules.
In conclusion, in the future the statistical model-based IR will be an indispensable alternative to FBP. It will improve the standard imaging methods, specifically for lung-cancer screening, and will remove drawbacks of FBP-based CT screening programs.
Acknowledgments
The authors thankfully acknowledge the grateful cooperation of the expert radiologists and medical engineers involved. CT scans were acquired with a Somatom Force CT installed at the Essen University Hospital.
Funding: None.
Footnote
Provenance and Peer Review: This article was commissioned by the Guest Editors (Wieland Voigt and Helmut Prosch) for the series “Lung Cancer Screening” published in Shanghai Chest. The article has undergone external peer review.
Data Sharing Statement: Available at https://dx.doi.org/10.21037/shc-21-9
Conflicts of Interest: All authors have completed the ICMJE uniform disclosure form (available at https://dx.doi.org/10.21037/shc-21-9). The series “Lung Cancer Screening” was commissioned by the editorial office without any funding or sponsorship. The authors have no other conflicts of interest to declare.
Ethical Statement: The authors are accountable for all aspects of the work in ensuring that questions related to the accuracy or integrity of any part of the work are appropriately investigated and resolved. The procedures performed in study involved phantoms, but no human participants and no animals.
Open Access Statement: This is an Open Access article distributed in accordance with the Creative Commons Attribution-NonCommercial-NoDerivs 4.0 International License (CC BY-NC-ND 4.0), which permits the non-commercial replication and distribution of the article with the strict proviso that no changes or edits are made and the original work is properly cited (including links to both the formal publication through the relevant DOI and the license). See: https://creativecommons.org/licenses/by-nc-nd/4.0/.
References
- Celeng C, Takx RAP, Lessmann N, et al. The Association Between Marital Status, Coronary Computed Tomography Imaging Biomarkers, and Mortality in a Lung Cancer Screening Population. J Thorac Imaging 2020;35:204-9. [Crossref] [PubMed]
- National Lung Screening Trial Research Team. Reduced lung-cancer mortality with low-dose computed tomographic screening. N Engl J Med 2011;365:395-409. [Crossref] [PubMed]
- National Lung Screening Trial Research Team. Results of initial low-dose computed tomographic screening for lung cancer. N Engl J Med 2013;368:1980-91. [Crossref] [PubMed]
- De Koning H, Van Der Aalst C, Ten Haaf K, et al. Effects of volume CT lung cancer screening: Mortality results of the NELSON randomized-controlled population based trial. J Thorac Oncol 2018;13:185. [Crossref]
- de Koning HJ, van der Aalst CM, de Jong PA, et al. Reduced Lung-Cancer Mortality with Volume CT Screening in a Randomized Trial. N Engl J Med 2020;382:503-13. [Crossref] [PubMed]
- Han D, Heuvelmans MA, Vliegenthart R, et al. An Update on the European Lung Cancer Screening Trials and Comparison of Lung Cancer Screening Recommendations in Europe. J Thorac Imaging 2019;34:65-71. [Crossref] [PubMed]
- Oudkerk M, Devaraj A, Vliegenthart R, et al. European position statement on lung cancer screening. Lancet Oncol 2017;18:e754-66. [Crossref] [PubMed]
- Becker N, Motsch E, Gross ML, et al. Randomized study on early detection of lung cancer with MSCT in Germany: study design and results of the first screening round. J Cancer Res Clin Oncol 2012;138:1475-86. [Crossref] [PubMed]
- Wille MM, Dirksen A, Ashraf H, et al. Results of the Randomized Danish Lung Cancer Screening Trial with Focus on High-Risk Profiling. Am J Respir Crit Care Med 2016;193:542-51. [Crossref] [PubMed]
- Yousaf-Khan U, van der Aalst C, de Jong PA, et al. Final screening round of the NELSON lung cancer screening trial: the effect of a 2.5-year screening interval. Thorax 2017;72:48-56. [Crossref] [PubMed]
- Kauczor HU, Baird AM, Blum TG, et al. ESR/ERS statement paper on lung cancer screening. Eur Respir J 2020;55:1900506 [Crossref] [PubMed]
- Mascalchi M, Sali L. Lung cancer screening with low dose CT and radiation harm-from prediction models to cancer incidence data. Ann Transl Med 2017;5:360. [Crossref] [PubMed]
- Nanavaty P, Alvarez MS, Alberts WM. Lung cancer screening: advantages, controversies, and applications. Cancer Control 2014;21:9-14. [Crossref] [PubMed]
- O'Dowd EL, Baldwin DR. Lung cancer screening-low dose CT for lung cancer screening: recent trial results and next steps. Br J Radiol 2018;91:20170460 [Crossref] [PubMed]
- Hunger T, Wanka-Pail E, Brix G, et al. Lung Cancer Screening with Low-Dose CT in Smokers: A Systematic Review and Meta-Analysis. Diagnostics (Basel) 2021;11:1040. [Crossref] [PubMed]
- U.S. Preventive Services Task Force, March 09, 2021. Available online: www.uspreventiveservicestaskforce.org
- Siemens Healthineers, Erlangen, Germany. Available online: https://www.siemens-healthineers.com/
- Willemink MJ, Noël PB. The evolution of image reconstruction for CT-from filtered back projection to artificial intelligence. Eur Radiol 2019;29:2185-95. [Crossref] [PubMed]
- König B, Guberina N, Kühl H, et al. Design and first results of a phantom study on the suitability of iterative reconstruction for lung-cancer screening with low-dose computer tomography. Current Directions in Biomedical Engineering 2019;5:593-6. [Crossref]
- König B, Guberina N, Kühl H, et al. Validation of iterative CT reconstruction by inter and intra observer performance assessment of artificial lung foci. Current Directions in Biomedical Engineering 2020; [Crossref]
- König B, Gernhardt C, Guberina N, et al. Suitability of iterative reconstruction for lung-cancer screening with low-dose computer tomography: First results of a phantom study. European Society of Radiology, 2020. doi:
10.26044/ecr2020/C-02150 .10.26044/ecr2020/C-02150 - Wielpütz MO, Wroblewski J, Lederlin M, et al. Computer-aided detection of artificial pulmonary nodules using an ex vivo lung phantom: influence of exposure parameters and iterative reconstruction. Eur J Radiol 2015;84:1005-11. [Crossref] [PubMed]
- Fu B, Wang G, Wu M, et al. Influence of CT effective dose and convolution kernel on the detection of pulmonary nodules in different artificial intelligence software systems: A phantom study. Eur J Radiol 2020;126:108928 [Crossref] [PubMed]
- Blazis SP, Dickerscheid DBM, Linsen PVM, et al. Effect of CT reconstruction settings on the performance of a deep learning based lung nodule CAD system. Eur J Radiol 2021;136:109526 [Crossref] [PubMed]
- Chamberlin J, Kocher MR, Waltz J, et al. Automated detection of lung nodules and coronary artery calcium using artificial intelligence on low-dose CT scans for lung cancer screening: accuracy and prognostic value. BMC Med 2021;19:55. [Crossref] [PubMed]
- Kalender WA. Computed Tomography. 2nd edition. New York: Wiley-VCH, 2005.
- Flohr TG, Stierstorfer K, Ulzheimer S, et al. Image reconstruction and image quality evaluation for a 64-slice CT scanner with z-flying focal spot. Med Phys 2005;32:2536-47. [Crossref] [PubMed]
- Kyriakou Y, Kachelriess M, Knaup M, et al. Impact of the z-flying focal spot on resolution and artifact behavior for a 64-slice spiral CT scanner. Eur Radiol 2006;16:1206-15. [Crossref] [PubMed]
- Kalender WA, Perman WH, Vetter JR, et al. Evaluation of a prototype dual-energy computed tomographic apparatus. I. Phantom studies. Med Phys 1986;13:334-9. [Crossref] [PubMed]
- Lell MM, Kachelrieß M. Recent and Upcoming Technological Developments in Computed Tomography: High Speed, Low Dose, Deep Learning, Multienergy. Invest Radiol 2020;55:8-19. [Crossref] [PubMed]
- QRM GmbH, Moehrendorf, Germany. Available online: www.qrm.de
- Kyoto Kagaku Co.,Ltd., Kyoto, Japan. Available online: www.kyotokagaku.com
- Miller AR, Jackson D, Hui C, et al. Lung nodules are reliably detectable on ultra-low-dose CT utilising model-based iterative reconstruction with radiation equivalent to plain radiography. Clin Radiol 2019;74:409.e17-22. [Crossref] [PubMed]
Cite this article as: König B, Kühl H, Zylka W. Computed tomography lung-cancer screening: does iterative reconstruction matter? Shanghai Chest 2021;5:35.